Latest research conducted by a team at Baylor College of Medicine has proposed that an innovative strategy may hold the possibility to give an early diagnosis of schizophrenia. The strategy applied a machine learning algorithm called SPLS-DA to analyze specific regions of the human genome called CoRSIVs, hoping to reveal epigenetic markers for the condition.
DNA samples were drawn from patients of schizophrenia and people without the disease. Testing confirmed that in the DNA samples of schizophrenics, epigenetic markers were found, a profile of methyl chemical groups was identified. This difference is what helped develop the model and would later be used to assess an individual’s probability of having the condition. Testing the model on an independent dataset revealed that it can identify schizophrenia patients with 80% accuracy. The study appears in the journal Translational Psychiatry.
“Schizophrenia is a devastating disease that affects about 1% of the world’s population,” said corresponding author Dr. Robert A. Waterland professor of pediatrics – nutrition at the USDA/ARS Children’s Nutrition Research Center at Baylor and of molecular and human genetics. “Although genetic and environmental components seem to be involved in the condition, current evidence only explains a small portion of cases, suggesting that other factors, such as epigenetic, also could be important.”
To address this obstacle, Waterland and his colleagues had identified in previous work a set of specific genomic regions in which DNA methylation, a common epigenetic marker, differs between people but is consistent across different tissues in one person. They called these genomic regions CoRSIVs for correlated regions of systemic interindividual variation. They proposed that studying CoRSIVs is a novel way to uncover epigenetic causes of disease.
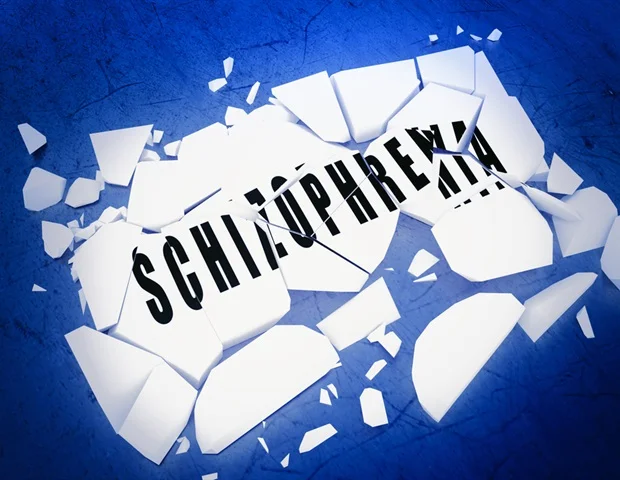
“Because methylation patterns in CoRSIVs are the same in all the tissues of one individual, we can analyze them in a blood sample to infer epigenetic regulation on other parts of the body that are difficult to assess, such as the brain,” Waterland said.
Many previous studies have analyzed methylation profiles in blood samples in order to identify epigenetic differences between individuals with schizophrenia, the researchers explained.
“Our study is innovative in various ways,” claimed the first author Dr. Chathura J. Gunasekara, computer scientist in the Waterland lab. “We focused on CoRSIVs and also applied for the first time the SPLS-DA machine learning algorithm to analyze DNA methylation. As a scientist interested in applying machine learning to medicine, our findings are very exciting. They not only suggest the possibility of predicting risk of schizophrenia early in life, but also outline a new approach that may be applicable to other diseases.”
The study is also considered innovative as it has taken into account factors that were previously left untouched. For instance, methylation patterns in blood can be affected by factors such as smoking and taking antipsychotic medications, both of which are common in schizophrenia patients.
“Here, we took various approaches to evaluate whether the methylation patterns we detected at CoRSIVs were affected by medication use and smoking. We were able to rule that out,” Waterland said. “This, together with the fact that DNA methylation at CoRSIVs is established very early in life, indicates that the epigenetic differences we identified between schizophrenia patients and healthy individuals were there before the disease was diagnosed, suggesting they may contribute to the condition.”
Using this approach, the researchers were able to achieve much stronger epigenetic signals associated with schizophrenia than has ever been done before, said the team.
“We consider our study a proof of principle that focusing on CoRSIVs makes epigenetic epidemiology possible,” Waterland said.